2021.beamsummit.org/sessions/lessons-learned-dataflow-ml-batch-inference
Preview meta tags from the 2021.beamsummit.org website.
Linked Hostnames
9- 10 links to2021.beamsummit.org
- 2 links tobeam.apache.org
- 1 link to2020.beamsummit.org
- 1 link toeurope2019.beamsummit.org
- 1 link tona2019.beamsummit.org
- 1 link tosessionize.com
- 1 link totwitter.com
- 1 link towww.linkedin.com
Thumbnail
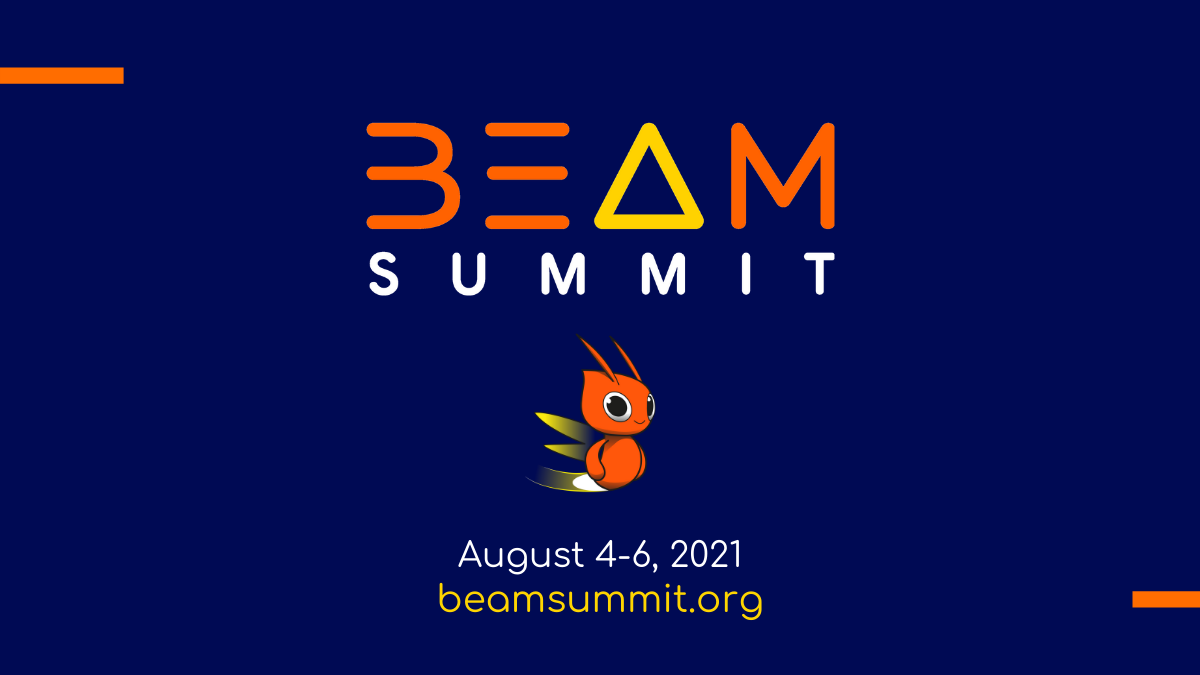
Search Engine Appearance
https://2021.beamsummit.org/sessions/lessons-learned-dataflow-ml-batch-inference
Lessons learned from using Dataflow for local ML batch inference
The Beam Summit brings together experts and community to share the exciting ways they are using, changing, and advancing Apache Beam and the world of data and stream processing.
Bing
Lessons learned from using Dataflow for local ML batch inference
https://2021.beamsummit.org/sessions/lessons-learned-dataflow-ml-batch-inference
The Beam Summit brings together experts and community to share the exciting ways they are using, changing, and advancing Apache Beam and the world of data and stream processing.
DuckDuckGo
Lessons learned from using Dataflow for local ML batch inference
The Beam Summit brings together experts and community to share the exciting ways they are using, changing, and advancing Apache Beam and the world of data and stream processing.
General Meta Tags
8- titleLessons learned from using Dataflow for local ML batch inference | Beam Summit 2021
- charsetutf-8
- viewportwidth=device-width,initial-scale=1,maximum-scale=1
- descriptionThe Beam Summit brings together experts and community to share the exciting ways they are using, changing, and advancing Apache Beam and the world of data and stream processing.
- authorSoftware Guru
Open Graph Meta Tags
5- og:titleLessons learned from using Dataflow for local ML batch inference
- og:descriptionAt BenchSci, we mine the world’s biological research papers with the aim of extracting information that will accelerate future pharmaceutical research programs by enabling more reproducible experiments. Machine Learning and specifically our in-house Deep Learning models play an important role in extracting these key pieces of information and organizing the knowledge into a meaningful and easy-to-use structure. While we have the luxury of processing this information in batch, the size and number of our models along with the size of the input data eventually outgrew our on-premise model serving infrastructure.
- og:typearticle
- og:urlhttps://2021.beamsummit.org/sessions/lessons-learned-dataflow-ml-batch-inference/
- og:imagehttps://2021.beamsummit.org/images/card.png
Twitter Meta Tags
4- twitter:cardsummary_large_image
- twitter:imagehttps://2021.beamsummit.org/images/card.png
- twitter:titleLessons learned from using Dataflow for local ML batch inference
- twitter:descriptionAt BenchSci, we mine the world’s biological research papers with the aim of extracting information that will accelerate future pharmaceutical research programs by enabling more reproducible experiments. Machine Learning and specifically our in-house Deep Learning models play an important role in extracting these key pieces of information and organizing the knowledge into a meaningful and easy-to-use structure. While we have the luxury of processing this information in batch, the size and number of our models along with the size of the input data eventually outgrew our on-premise model serving infrastructure.
Link Tags
6- icon/favicon.ico
- shortcut icon/favicon.ico
- stylesheet/plugins/bootstrap/css/bootstrap.min.css
- stylesheet/plugins/themefisher-font/themefisher-font.min.css
- stylesheethttps://unpkg.com/[email protected]/dist/bootstrap-table.min.css
Emails
1Links
19- https://2020.beamsummit.org
- https://2021.beamsummit.org
- https://2021.beamsummit.org/about
- https://2021.beamsummit.org/coc
- https://2021.beamsummit.org/faq