www.nature.com/articles/s41598-024-76719-w
Preview meta tags from the www.nature.com website.
Linked Hostnames
39- 150 links towww.nature.com
- 32 links toarxiv.org
- 26 links todoi.org
- 10 links toscholar.google.com
- 7 links towww.springernature.com
- 6 links towww.ncbi.nlm.nih.gov
- 4 links topartnerships.nature.com
- 3 links toauthorservices.springernature.com
Thumbnail
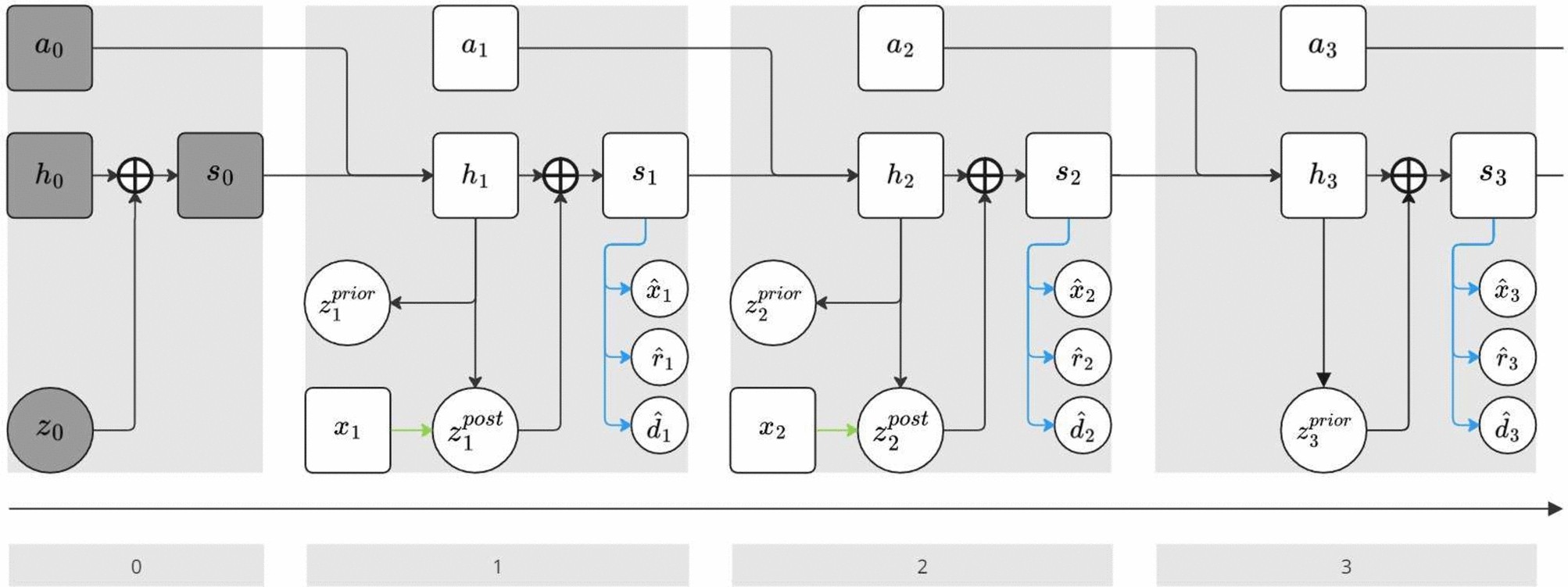
Search Engine Appearance
Exploring the limits of hierarchical world models in reinforcement learning - Scientific Reports
Hierarchical model-based reinforcement learning (HMBRL) aims to combine the sample efficiency of model-based reinforcement learning with the abstraction capability of hierarchical reinforcement learning. While HMBRL has great potential, the structural and conceptual complexities of current approaches make it challenging to extract general principles, hindering understanding and adaptation to new use cases, and thereby impeding the overall progress of the field. In this work we describe a novel HMBRL framework and evaluate it thoroughly. We construct hierarchical world models that simulate the environment at various levels of temporal abstraction. These models are used to train a stack of agents that communicate top-down by proposing goals to their subordinate agents. A significant focus of this study is the exploration of a static and environment agnostic temporal abstraction, which allows concurrent training of models and agents throughout the hierarchy. Unlike most goal-conditioned H(MB)RL approaches, it also leads to comparatively low dimensional abstract actions. Although our HMBRL approach did not outperform traditional methods in terms of final episode returns, it successfully facilitated decision-making across two levels of abstraction. A central challenge in enhancing our method’s performance, as uncovered through comprehensive experimentation, is model exploitation on the abstract level of our world model stack. We provide an in depth examination of this issue, discussing its implications and suggesting directions for future research to overcome this challenge. By sharing these findings, we aim to contribute to the broader discourse on refining HMBRL methodologies.
Bing
Exploring the limits of hierarchical world models in reinforcement learning - Scientific Reports
Hierarchical model-based reinforcement learning (HMBRL) aims to combine the sample efficiency of model-based reinforcement learning with the abstraction capability of hierarchical reinforcement learning. While HMBRL has great potential, the structural and conceptual complexities of current approaches make it challenging to extract general principles, hindering understanding and adaptation to new use cases, and thereby impeding the overall progress of the field. In this work we describe a novel HMBRL framework and evaluate it thoroughly. We construct hierarchical world models that simulate the environment at various levels of temporal abstraction. These models are used to train a stack of agents that communicate top-down by proposing goals to their subordinate agents. A significant focus of this study is the exploration of a static and environment agnostic temporal abstraction, which allows concurrent training of models and agents throughout the hierarchy. Unlike most goal-conditioned H(MB)RL approaches, it also leads to comparatively low dimensional abstract actions. Although our HMBRL approach did not outperform traditional methods in terms of final episode returns, it successfully facilitated decision-making across two levels of abstraction. A central challenge in enhancing our method’s performance, as uncovered through comprehensive experimentation, is model exploitation on the abstract level of our world model stack. We provide an in depth examination of this issue, discussing its implications and suggesting directions for future research to overcome this challenge. By sharing these findings, we aim to contribute to the broader discourse on refining HMBRL methodologies.
DuckDuckGo
Exploring the limits of hierarchical world models in reinforcement learning - Scientific Reports
Hierarchical model-based reinforcement learning (HMBRL) aims to combine the sample efficiency of model-based reinforcement learning with the abstraction capability of hierarchical reinforcement learning. While HMBRL has great potential, the structural and conceptual complexities of current approaches make it challenging to extract general principles, hindering understanding and adaptation to new use cases, and thereby impeding the overall progress of the field. In this work we describe a novel HMBRL framework and evaluate it thoroughly. We construct hierarchical world models that simulate the environment at various levels of temporal abstraction. These models are used to train a stack of agents that communicate top-down by proposing goals to their subordinate agents. A significant focus of this study is the exploration of a static and environment agnostic temporal abstraction, which allows concurrent training of models and agents throughout the hierarchy. Unlike most goal-conditioned H(MB)RL approaches, it also leads to comparatively low dimensional abstract actions. Although our HMBRL approach did not outperform traditional methods in terms of final episode returns, it successfully facilitated decision-making across two levels of abstraction. A central challenge in enhancing our method’s performance, as uncovered through comprehensive experimentation, is model exploitation on the abstract level of our world model stack. We provide an in depth examination of this issue, discussing its implications and suggesting directions for future research to overcome this challenge. By sharing these findings, we aim to contribute to the broader discourse on refining HMBRL methodologies.
General Meta Tags
138- titleExploring the limits of hierarchical world models in reinforcement learning | Scientific Reports
- titleClose banner
- titleClose banner
- X-UA-CompatibleIE=edge
- applicable-devicepc,mobile
Open Graph Meta Tags
5- og:urlhttps://www.nature.com/articles/s41598-024-76719-w
- og:typearticle
- og:site_nameNature
- og:titleExploring the limits of hierarchical world models in reinforcement learning - Scientific Reports
- og:imagehttps://media.springernature.com/m685/springer-static/image/art%3A10.1038%2Fs41598-024-76719-w/MediaObjects/41598_2024_76719_Fig1_HTML.jpg
Twitter Meta Tags
6- twitter:site@SciReports
- twitter:cardsummary_large_image
- twitter:image:altContent cover image
- twitter:titleExploring the limits of hierarchical world models in reinforcement learning
- twitter:descriptionScientific Reports - Exploring the limits of hierarchical world models in reinforcement learning
Item Prop Meta Tags
5- position1
- position2
- position3
- position4
- publisherSpringer Nature
Link Tags
15- alternatehttps://www.nature.com/srep.rss
- apple-touch-icon/static/images/favicons/nature/apple-touch-icon-f39cb19454.png
- canonicalhttps://www.nature.com/articles/s41598-024-76719-w
- icon/static/images/favicons/nature/favicon-48x48-b52890008c.png
- icon/static/images/favicons/nature/favicon-32x32-3fe59ece92.png
Emails
1Links
279- http://adsabs.harvard.edu/cgi-bin/nph-data_query?link_type=ABSTRACT&bibcode=2003JOSAA..20.1434L
- http://adsabs.harvard.edu/cgi-bin/nph-data_query?link_type=ABSTRACT&bibcode=2019Natur.575..350V
- http://arxiv.org/abs/1105.1186
- http://arxiv.org/abs/1312.5602
- http://arxiv.org/abs/1509.02971